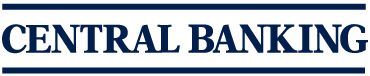
Big data in central banks: 2018 survey results
As work in big data enters the mainstream for central banks, its policymaking and supervisory influence is expanding, prompting significant investment in new technologies.
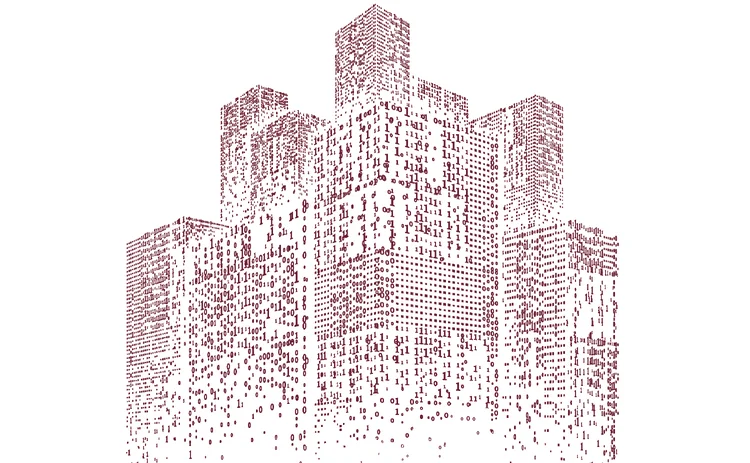
This new survey – the third in a series conducted by Central Banking in association with BearingPoint1 – reports on the approach central banks take towards big data, and data management more broadly. This report has only been possible with the support and co-operation of the central bankers who agreed to take part. They did so on the condition that neither their names nor those of their central banks would be disclosed in this report.
Key findings
- Work in big data can now be considered a mainstream activity for central banks: over half of survey respondents said they are working on a big data project.
- Big data plays an increasingly significant role in policymaking and supervisory processes. More than 60% of respondents said big data was either a “core input” or an “auxiliary input” into policymaking, an increase on last year’s survey.
- Data governance remains a work in progress for central banks. More than 60% of respondents do not believe their central bank has a clear structure for data governance.
- Spending on data in central banks is decentralised. More than 80% of respondents do not have a single allocated budget for the handling of data.
- Central banks tend to focus their big data investments on software and hardware over human resources and security.
- Ensuring sufficient quality is seen as the greatest challenge when collecting and managing data.
- Central banks increasingly look to external sources to obtain big data.
- Central banks typically make use of a combination of methods to process regulatory data collection. The most popular combination is a self-developed data platform, Excel and data-based handling.
- Central banks are increasingly bringing in new external technologies to manage data. Just under 40% of respondents said they had done this in the past 12 months.
- New technology has yet to exert a significant impact on central banks’ supervisory architecture.
- Central banks see monetary policy as standing to benefit most from big data.
- Central banks typically use a range of methodological approaches to analyse big data, with data mining being the most popular.
- Big data is established as a tool for forecasting and nowcasting.
Profile of respondents
Survey questionnaires were sent to 130 central banks in May 2018. By June, responses had been received from 52 central banks.2 The average staff size was 2,205 – slightly below the industry average – and 31 respondents had fewer than 1,000 employees. Just over 40% of respondents were from emerging‑market countries, and the most popular source of responses was statistics departments, which accounted for nearly two-thirds.

Percentages in some tables may not total 100 due to rounding.
Is your central bank working on a project involving big data?

It is a time of change for central banks and big data. Work involving big data can now be considered a mainstream activity: more than half of survey respondents said their central bank was working on a project in this area. For some central banks, this is a relatively new endeavour and their objectives are purely research-based, exploring prototypes and concepts. Other central banks are more advanced, examining how big data can influence policy.
The 29 central banks working on a project involving big data were drawn from across the economic spectrum, but industrial countries figured prominently, and more than half of the group were larger central banks – those with more than 1,000 members of staff. In their comments, several respondents described sophisticated frameworks in place for big data development.
A European central bank detailed the progress of its projects over the past two years: “In 2016, the statistics department launched the ‘big data forum’, which is intended to be for internal discussion. In roundtables, a team with specialists in different statistical domains tries to identify improvements to the statistics it produces using ‘unconventional’ sources of information and in that way encourages the development of big data projects. In 2017, the data integration and sharing unit was created.”
Similarly, a statistician at a large institution commented on several advances it had made: “We are working in different areas related to big data. We have conducted different projects using information obtained from Google Trends to improve forecasting. A project is also in progress analysing texts as a proxy for confidence indicators. We have used machine learning (random forests) in a project to measure the effects of fiscal anticipation in exploiting texts from television news. There is also an ongoing project in the field of media economics that uses unsupervised learning and intensive use of automatic text analysis. Moreover, some problems have been tackled using ‘big data techniques’ (such as neuronal networks) even if no big data as such has been used. Likewise, machine-learning techniques are being used to solve dynamic stochastic general equilibrium models with heterogeneous agents. More recently, a couple of initiatives have been started: one with web scraping to improve the information we have on the housing market, and another using machine learning to improve the quality of statistics.”
The dynamic nature of the field is highlighted by the novelty of the work: more than half of the 29 central banks reported that their projects were less than a year old. A respondent from a large central bank was testing the waters in this area with a prototype: “As part of our granular data model, we started a proof of concept to validate granular data.” The largest central bank reported that several projects are in progress.
Comments also indicated that administrative data – data collected via the work of governments and other official sector institutions – is a beneficial source for central banks starting out in the field. A respondent from the Americas noted their central bank’s data sources: “Tax information, which is used to compute a monthly activity index and yearly GDP.” A large central bank in Asia was interested in real estate developments: “Currently, we are using web-based and administrative data to understand developments in the property market.”
A central bank from a developing economy was keen to ensure data quality: “The central bank is working to optimise financial market statistics and monetary statistics in order to reduce duplication and contradictions in the data collected.” The largest institution in this survey commented that it was working on “loan loss risk analysis and big data collection for the financial institution’s supervision.”
Has this project been initiated in the past 12 months?

For 13 central banks, these projects have been in process for a considerable period of time. These central banks tended to be smaller – 10 had fewer than 1,000 head of staff. The duration of these projects ranged from just over one year to as much as 10 years. An industrial-economy central bank commented: “This line of action has been active for some years, involving new and already existing data sources and business requirements.”
Twenty‑three central banks – 45% of respondents – are not involved in big data projects. This group largely comprised emerging-market central banks. In their comments it was clear that, for some, big data is an area of interest for the future, with several indicating work would soon start.
Which best represents your central bank’s view of big data?

Big data is set to play an increasingly influential role in policymaking and supervisory processes. More than 60% of respondents said big data was either a “core input” or an “auxiliary input” into policymaking – an increase on the results of last year’s survey.3 Interestingly, the number of central banks that see it as an auxiliary input into policymaking is almost double that of last year, while the proportion that sees it as an active “area of research” has decreased three percentage points from the 2017 survey figure of 42%.
A central bank based in Oceania said: “Data captured should ultimately be a core input for policymaking and supervisory processes.” Administrative data is widely seen as an auxiliary input: “The central bank has a well-organised statistical business process model. So our database includes large amounts of information necessary for monetary and financial statistics production in line with the international standards. However, in some analytical materials, we use administrative data and other sources as an auxiliary input.”
Of those that see big data as a core input, half are from developing‑economy countries, with all of these institutions smaller than the survey average. Staff numbers on average were higher for the 19 central banks treating big data as an auxiliary input. In Asia, a central bank noted that its projects were focused on nowcasting: “We have used big data for nowcasting GDP, but we still need other traditional models or methods to forecast its long‑term trend.”
Twenty central banks said big data is an active area of research, with respondents from larger institutions typically holding this view. Many reasoned the projects were still in preliminary stages, and therefore big data was too underdeveloped to be used in policymaking. Several comments received, however, suggest this will change over time. A central banker from Europe said: “The central bank’s big data projects are mostly still at the research stage. If successful, they may become an auxiliary input into policymaking.”
Central banks’ views of big data and its role in policymaking has not generally shifted in the past 12 months; 90% of respondents said their view was unchanged.
Does your central bank have a clear data governance structure for the management and collection of data?

Data governance remains a work in progress for central banks. More than 60% of respondents said their central bank – in their view – does not have a clear structure for data governance. This finding indicates a slight increase on last year’s survey. It is interesting to compare those with the ambition to use big data for policymaking purposes (61% of respondents) and their governance structure. Of the 61%, half do not have a clear data governance structure.
Of the 32 central banks that do not have a clear data governance structure in place, two-thirds are from emerging-market countries and are typically smaller institutions. Comments from these respondents can be broadly divided into three categories: employing a decentralised strategy, placing the responsibility solely with the statistics department and outsourcing the process. A central bank in Europe fell into the first category: “The central bank pursues, at the moment, a bottom‑up strategy. We have clear roles and responsibilities for each dimension of data governance, such as data quality and IT security in the responsible divisions.” A central banker from the Americas similarly commented: “Data governance is decentralised. Expertise is spread across departments with focal points in different departments.” A central banker in Africa added: “Each department has its own personnel and methodology of keeping/storing data.”
The second cohort noted that their statistics departments alone were responsible for big data projects, as this is their remit. Three central banks typified this sentiment in their comments. A small central bank said: “The central bank co-ordinates its statistical activities and data collections through its statistics department.” Equally, a Europe‑based central bank commented that its “statistics and reporting department is responsible for data management, but we do not have a clear structure.”
Outsourcing data management – the preference of the third group – was described by a statistician from a large central bank in Europe: “The initial data processing is procured and outsourced.”
Four central banks noted they are in the process of developing a governance structure. An African respondent said that their central bank is “currently working on [an] expanded structure to integrate statistics and other department stakeholders.”
At central banks with a governance structure, some respondents highlighted the novelty of this, and described how they look to prioritise and support big data projects by restructuring departments and maximising input to central bank policy. Implementation was a relatively new endeavour for two central banks in Europe; one introduced a governance structure last year: “In June 2017, the bank established the data governance committee chaired by the chief data officer and the first deputy of the bank.” The second example was put into place earlier this year: “As of April 2018, a new section has been formed to facilitate more effective data sharing and data analytics within the central bank.”
A central bank with just over 1,500 members of staff made considerable changes to accommodate big data developments: “The central bank has a governance structure for analytic data that supports its monetary policy and financial system functions. This includes a central data and statistics office and an analytic environment governance committee that oversees the use of financial resources to acquire data, software, hardware and to fund big data projects, among other things.”
The establishment of clear data governance structure has led one European central bank to reassess the roles and responsibilities of its employees: “The central bank has a clear role division between employees throughout the data phase: collection, processing, analysis and storage. Functions according to the role distribution are approved in job descriptions.”
Is the data governance gap widening?
Three years of surveying central banks on big data has highlighted a gap between those that do not see their data governance as clear and those that do. This gap appears to be widening. In 2016, those who felt governance was not clear held the slimmest of majorities (see chart below). In 2017 this majority increased to seven and, in 2018, is more than 10. Fewer than 40% see their data governance as clear. So the immediate questions are: why? And: does it matter?
Taking the latter first, it is difficult to answer “no”, certainly given the interest and activity in big data and the importance central banks place in governance. So, while one can commend respondents for their honesty, from a policy perspective the trend is heading in the wrong direction.
Second, to the “why?”. This is harder, not least as the composition of survey respondents has changed over the three years. But, from comments to this question – and others – one can imagine cases where initiatives, policy interest and activity are running ahead of structure and management. Big data cuts across departments, divisions and policy. Quite simply, it is disrupting central banking, and changing governance structures to meet that disruption takes time and effort.
Where governance is clear, as an extended comment this year reveals, the structure reflects these rich seams of interest, but assigns clear responsibility at departmental level, with a single person responsible for overall co-ordination: “The bank has an integrated model of information management based on an information governance structure, which attributes the responsibility of operational management, in co-ordination with the IT department, to the statistics department. Each department of the bank has a data steward responsible for the content and management of the data it produces. There is also a master data steward, who co‑ordinates the activity and oversees general guidelines.”
Does your central bank have a clear data governance structure for the management and collection of data?

Does your central bank have a single allocated budget for the handling of data – including big data?

Spending on data in central banks is, for the most part, decentralised. More than 80% of respondents do not have an allocated budget for handling data. In their comments, central bankers explained that the budget for data was either allocated per division/department or per project.
Two central banks have no assigned budget as the tasks are carried out by employees as part of their day-to-day work. This was the case for a central bank in Africa: “The bank employs statisticians and assistant statisticians who are mainly responsible for the collection – including management of database – and dissemination of data.”
Less than one in five respondents said their central bank has an allocated budget for data handling. Almost all of these nine central banks were small institutions with fewer than 1,000 members of staff and were from emerging‑market and developing countries. Only two submitted comments, noting that their budgets were specifically allocated to their IT departments. The $100,000 budget of a respondent from Asia-Pacific included operational resources: “This budget is allocated to the IT unit, which is a part of the currency and corporate services group. This amount includes software and hardware.”
The budget is assigned annually for one European central bank and is predominantly used for technical support: “For each IT system, a budget is established for the technical support of the system on an annual basis. Technical support includes database licences, operating systems, hardware support and telephone support for users.”
For many central banks, the allocation of departmental budgets takes priority over the assignment of a big data budget. The onus therefore shifts to departments to assign an appropriate budget to big data projects. At one Middle East central bank, department needs hold sway: “Data handling is resourced based on the needs of the internal departments that handle their own data specific to their functions.”
In what sector does your central bank focus big data investment?

Central banks focus big data investment on software and hardware over human resources and security. Improving software for data management and analysis tools is a high priority for respondents. A respondent from the 85% of central banks that invest in software commented on investments currently under way: “There is a data warehouse and business intelligence project that is ongoing. The focus of this has been on data acquisition and preparation for analysis and insight.”
Central banks are also keen to improve hardware. Most of the 64% in this category are small institutions from emerging-market economies. For a central bank in Oceania, processing power is essential to ensure advances in big data: “Storage and processing power has always been an area of priority to maintain and manage data growth; however, recently the bank has invested in a security information and event management solution, which manages, correlates and reports on security activities. The bank also focuses on in-house application development, which is a tool for data management and analysis.”
Human resources investment is a somewhat lower priority. Forty‑four per cent of central banks focus on this area – one-third of which were from small central banks in industrial economies. Likewise, security was the choice of 44% of respondents. These were relatively small central banks, with fewer than 2,000 members of staff. Security was a particular issue for European central banks, with one respondent noting: “Investments in security to allow storage of more sensitive data are likely to happen in the next few years.”
Does your central bank collect and manage granular and/or raw data?

Central banks typically make use of a combination of data types from different data sources in big data projects, although there is a marginal preference for granular data over raw data. Granular data is detailed data or the lowest level of data within a set, while raw data is unprocessed data.
Thirty‑one central banks collect and manage both raw and granular data. This combination was a particularly popular choice for emerging-market and developing economies. A European central banker’s comment typified it: “The central bank collects and manages raw data, as well as granular data.” Another European central bank that collects and manages both raw and granular data commented: “We collect financial and statistical data from financial institutions only. Only a few amounts of data are obtained from other institutions such as public registers, national statistics offices and ministries of finance.”
Financial institutions featured significantly in comments made by respondents, and were exemplified by one central banker: “The bank collects different types of micro-data, mainly from financial institutions and non-financial firms such as individual loans, security-by-security information, foreign transactions or firms’ balance sheets.”
Thirteen central banks source only granular data. Central banks from the Americas dominated this group. Indeed, over half of respondents that use granular data operate in an emerging-market economy.
Thirty-seven central banks – two-thirds from institutions smaller than the survey average – collect and manage raw data. Six collect and manage just raw data. For one African central bank, the bigger picture is sufficient for its big data projects: “The bank analyses and manages data at a higher level and currently there is no need to capture and manage granular data.”
What are the biggest challenges in collecting and managing granular data?

Data quality is widely seen as the greatest challenge central bankers face when collecting and managing granular data. Nineteen central banks – 46% of respondents – see this as most significant. In this category, developing and emerging-market economies were prevalent, particularly from Africa; however there was an even divide between small and large institutions. “Processing large volumes” ranked first or second for 48% of respondents, correlating with central banks’ increased investment in hardware and software – perhaps a reflection of concerns over having the right tools available.
The operational management of big data was an underlying priority, featuring as moderately important for most respondents. By contrast, “governance and budget” and “staff expertise and training” tended to be less of a concern, with 41 central banks ranking them second, third or fourth. This generally ranked higher for emerging-market central banks.
Varying levels of granularity is a relatively minimal challenge for central banks, with 62% of respondents placing it in fourth, fifth or sixth place. IT resources similarly ranked low as a concern, with 34% of respondents placing it in either fifth or sixth place.
Does your central bank obtain big data from external sources?

Central banks have traditionally been cautious about making use of third parties, but this may now be changing – at least with regard to data. Two‑thirds of respondents said they use external sources, a group dominated by small European central banks. Comments received from this group could be placed into three categories: sourcing external data from the internet, using administrative sources and employing commercial entities. The first category largely featured European central bankers: “Currently, our external sources are homepages available on the internet; no other external sources are being used.” A large European central bank commented: “We get some data from the internet (web searchers, web scraping, and so on) and also from news platforms.”
In the second category, admin-istrative data was a popular option, notably in developing and emerging-market economies. This is an ideal source for central banks in the early stages of big data development as it is easier to access and manage in comparison with some alternatives, such as internet sources. A respondent from Asia-Pacific commented: “The bank receives data from financial institutions, agencies, government, statutory bodies, superannuations and the private sector.” A central banker commented: “The central bank collects data from all supervised institutions, which are commercial banks, insurance companies, pension funds and microfinance institutions.”
A third set of comments mentioned commercial entities. These can be external technology providers that offer central banks tools and training to collect manage and analyse big data.
Seventeen central banks said they had yet to look at external sources for big data. Emerging markets made up over half of this group. “Sourcing external data has been a challenge. Data is usually submitted in semi- or fully processed form”, said a central banker from Africa. A European central banker said: “We get just aggregated data calculated from big data (for example, data of mobile operations).”
From which external sources does your central bank obtain big data?

Financial data is the primary source of big data, with more than 70% of respondents using it. Smaller institutions were in the majority here, with over three-quarters of respondents obtaining financial data having fewer than 1,000 head of staff. Industrial-economy central banks, on the other hand, look to expand and develop their resources, employing commercial entities for more complex datasets. Four central banks use these two options in combination, while nine central banks source financial and commercial data and one other alternative.
Social media datasets were the choice of relatively few, potentially due to being internet resources that require more expensive data processing.
How does your central bank process regulatory data collection?

Central banks typically employ a combination of methods to process regulatory data collection. The most popular combination among respondents is a self-developed data platform combined with Excel and data-based handling. As in previous iterations of Central Banking Journal’s big data
survey,1 self-developed data platforms are the most common method of data processing. This is a preferred option for emerging‑market economies and developing economies especially. European central banks are more likely to rely solely on self-developed data platforms.
Excel and data-based handling was identified as the method of choice for 28 central banks. This appears to be on the rise, with an increase of nine percentage points on the results from 2017. Developing countries and emerging-market countries were prevalent here, as were smaller central banks. However, few comments were offered.
Twenty-one central banks use commercial solutions to process regulatory data collection. A central banker from the Americas – a region that featured prominently in this category – noted the software/programs used: “Commercial solutions, Fame, CSDRMS.” A large industrial-economy central bank said: “The central bank does not have responsibility for supervision of commercial financial institutions, but does use regulatory data for analysis and to support input into policy decisions with supervisory entities.”
As in the 2016 survey, respondents made reference to Hadoop in their comments: “[For] the part of the work [that] is outsourced, data is processed in Hadoop.” At an African central bank, an alternative program is employed: “The bank has a solution called eFASS for collecting prudential returns and regulatory data from banks and other financial institutions.”
Has your central bank introduced any new technologies from the market to collect, prepare, check and/or analyse data in the past 12 months?

Central banks increasingly bring in new external technologies to manage data. Just under 40% of respondents reported that they had done this in the past 12 months. Comments from respondents showed that the new tools vary from automated systems and web portals to new formatting standards for reporting. The reasons given for this change were threefold: to enhance data quality, to save on staff resources and to cater for new datasets.
A European central bank reported on the introduction of its business model: “Recently, the national bank introduced a new innovative statistical business process model. It is intended for automation of data collection, validation and processing. It is an informational system that allows considerable optimisation of traditional statistical business process models.” From the respondents’ point of view, that means no paper or Excel spreadsheet reporting, nor overlapping and data inconsistency.
The largest institution to participate in the survey reported its new data format: “The XBRL format for report collection from non-banking financial institutions has been introduced.” Similarly, in Europe, a respondent was experimenting with granular data and subsequently created a prototype.
Thirty-one central banks said they had not added new data management technology in the past 12 months. This group was characterised by small central banks, with staff numbers below the survey average. Over half of these respondents were from emerging-market central banks. Nevertheless, developments are under way, as mentioned by three central banks from Africa, Europe and the Americas, respectively. Before investing in new technologies these central banks are assessing the best options for their institutions, as a European respondent explained: “The central bank is evaluating the purchase, installation and customisation of an integrated platform based on the cube structure methodology for the AnaCredit project.” An African central bank reported straightforwardly that “[a] project is under way to do this.”
Has your central bank adapted any of its supervisory processes to make use of new technologies, such as regtech and supervisory technology – or ‘suptech’ – in the past 12 months?

New technology has yet to exert a significant impact on central banks’ supervisory architectures. The few respondents to make changes were small institutions, with less than the survey average staff size. Comments made by respondents were in one of two categories: central banks in the process of implementation and central banks already employing new processes. The respondents in the latter category provided more detail of their work. A central banker from Africa noted: “GoAML for anti-money laundering (AML), Swift for sanctions screening.” Granular data played a role in the work of a European central bank: “We developed a granular data model, which is hosted by a joint venture of the banks.” Also in the second category, a central bank from the Middle East commented: “The centralised statistical system is a form of regtech and suptech, with ongoing improvements to supervisory processes to fully leverage its capabilities.”
Forty central bankers – 80% of respondents – said they had not made any changes to their processes in the past 12 months. In their comments, these central bankers expressed caution around making significant changes, but many had plans under way. One European central bank mentioned a new division had been formed to oversee financial innovations and market infrastructure.
Which areas stand to benefit most from big data?

Central bankers increasingly see monetary policy as standing to benefit most from big data. Twenty-two respondents to this year’s survey – exactly half – ranked this first, an increase on previous years.4 More than half of these respondents were from emerging-market economies and six from developing economies. Here, respondents noted the the primacy of monetary policy and, in some cases, the lack of micro-prudential responsibility at their institution. A smaller central bank from Europe saw work in the micro-prudential area as a project for the longer term: “The different applications that we now can see will aid both monetary policy and financial stability. The gains will be to further understand decisions made by firms and households, and to construct better indicators of firms’ and households’ behaviour. With time, perhaps [big data will] help in micro-supervision, but [this is] not likely in the short run.”
Around 90% of respondents prioritised macro-prudential policy first or second. Emerging-market central banks in particular highlighted macro-prudential policy, with five out of 27 prioritising it first, and 13 ranking it second. One-quarter of these respondents were representing large institutions with more than 2,000 members of staff.
Over half of respondents placed micro-prudential policy in third place. Just under half of these were from emerging-market economies, with industrial economies following closely with six respondents. A central bank that prioritised micro-prudential policy first commented: “While there is significant data available for monetary policy, there [is] maybe more potential to obtain supplementary information for banking supervision.”
Which of the following methodological approaches does your central bank use to analyse big data?

Central banks typically use a range of methodological approaches, with data mining being the most popular. Three-quarters of respondents checked multiple options, with the most popular combination being data mining and visual analytics – 10 respondents employ this approach.
Data mining was the most popular choice, with 23 respondents selecting this option. As a common first stage in big data projects, this is no great surprise. More than half of these respondents were from Europe, and the group was dominated by respondents based in emerging-market and industrial countries. Only three central banks mentioned data mining solely, while the remainder use it in combination with at least one other method.
Visual analytics allows the big data sourced to be more easily interpreted. Sixteen central banks use visual analytics to examine big data. Half of these were from the Americas. Only two central banks chose this option alone. Twelve central banks use trend forecasting to analyse big data, of which four use this alone – 11 of the 12 were from emerging markets.
Eleven respondents chose machine learning, of which just under half were from the Americas and Asia and the remainder from Europe. An Asian central bank using machine learning commented: “There are still many approaches that we need to learn from.” A European central bank that selected none of the above mentioned that this was a work in progress: “The bank does not currently analyse big data, but will be looking at tools such as machine learning and pattern recognition in the near future.” The same number – 11 – use pattern recognition. Developing economies comprise the majority here, with four central banks in total.
Selecting the “other” option, six central banks specified that they were working with “SQL queries, business objects”, “business intelligence tools” and “standard central bank indicators based on regulatory requirements, stress‑testing tools and other standard indicators”.
What does your central bank use big data for?

Big data is an established tool for economic projection. More than half of respondents said they used it for forecasting and nowcasting. Eighteen central banks use both of these; three use just one. An Asian central bank has been working with forecasting and nowcasting for some time: “We have been using big data for nowcasting and forecasting GDP since 2014.” A large European respondent was also investing in these tools; however its motivations differed: “[We use big data] for experimental purposes, and not as a policy tool.”
Stress testing was also popular, employed by 40% of respondents. An overwhelming majority in this group were small central banks with fewer than 2,000 staff. More than half of these respondents were from Africa and the Americas, with developing and emerging-market countries dominating.
Big data is emerging as a tool for risk mitigation. Nine central banks are using big data for cyber security, while 11 are using it for fraud detection/prevention and AML. From the comments, it was evident that many of these projects were still in the preliminary stages – if they were under way at all.
Does your central bank co-operate and co-ordinate its big data projects with any other institutions?

Co-operation and co-ordination of big data projects with other institutions is an ongoing process for central banks. Eighteen central banks – just over one-third of respondents – said they collaborate. This group featured central banks from all economic classifications but were mostly smaller institutions, with fewer than 2,000 employees. Comments tended to mention either the sharing of methodologies or the sharing of data sources.
Information and data were pooled from a variety of sources: committees and global institutions, national statistics offices and ministries of finance. At a European central bank, this was from the “Irving Fisher Committee and Eurostat.” In the Americas and Europe, “national statistics offices” were a popular source. For a central bank in Africa the International Monetary Fund (IMF) also provided support with data: “The bank consults/collaborates with the statistical service, ministry of finance and the IMF.” Universities were the go-to source for knowledge and advice for one Asian central bank:
“Our bank co-operates with university professors with regard to skills and big data management.”
Thirty-two central banks – a majority of respondents – do not currently collaborate on big data. These were drawn mainly from emerging-market and developing economies. The few comments that were submitted mentioned either that collaboration is under discussion or the central bank is not working on big data projects – the latter was given as a reason by three central banks.
What types of institution does your central bank co-operate with?

An overwhelming majority of central banks look to fellow national institutions when collaborating. A significant majority of central banks collaborate and co-operate with domestic state bodies – particularly ministries of finance and national statistics offices. Six central banks work with domestic bodies alone, and seven institutions collaborate with more than one other institution.
Has your central bank considered the legal and ethical limitations involved in big data processing and analytics in the past 12 months?

Data protection and, notably, the introduction of the European Union’s General Data Protection Regulation (GDPR) have prompted many central banks to consider the legal and ethical limitations involved in big data processing and analytics. Just over one-third – almost all from small institutions – said they had considered such issues. From the comments, it is clear the current climate of data protection is causing more central banks to consider the repercussions.
A respondent from the Americas noted: “This consideration is continuous as the bank eyes the advances made with the incorporation of big data globally. Recently, a cursory look at the requirements of the EU GDPR and its relationship to big data has been done as a part of information gathering as we explore opportunities for the inclusion of big data in the bank’s operations.”
A central bank in the Americas was of a similar view: “Data protection acts, as well as the EU’s GDPR legal implications, have been recently assessed by the central bank.”
For two central banks, the legal and ethical limitations are in line with their respective legal frameworks. A respondent from a transition economy noted: “We manage it by central bank procedures and rules.” An African central bank added: “Our legal department is involved in data architecture projects.” One large central bank is continuing to work on this issue: “Still to be developed as part of the learning process. This is also very much source dependent.”
Thirty-two central banks – two-thirds of which were from Europe, one-third from industrial economies – have not considered the legal and ethical limitations involved in big data processing. For a large central bank in the Americas, this was not a recent issue: “There have been some discussions at the bank about legal and ethical limitations, but nothing new in the past 12 months.” The question of source was a consideration for this European central bank, which did not regard legal and ethical limitations as a necessary consideration: “Our view is that unstructured data found on the internet is an “open source”. We do not handle any data on individuals.”
Notes
1. Emma Glass, Big data in central banking: 2016 survey; Central Banking, November 2016, www.centralbanking.com/2474825
Emma Glass, Big data in central banks: 2017 survey; Central Banking, November 2017, www.centralbanking.com/3315546
2. Of the 52 central banks that took part in this year’s survey, 35 also participated in last year’s. In total, 84 central banks have participated in the three surveys conducted so far.
3. While the samples come from different institutions, in 2017, 58% of respondents said big data was an input into policymaking.
4. Big data in central banking: 2016 survey, 18 central banks – 45% of respondents – thought monetary policy would benefit most.
Only users who have a paid subscription or are part of a corporate subscription are able to print or copy content.
To access these options, along with all other subscription benefits, please contact info@centralbanking.com or view our subscription options here: http://subscriptions.centralbanking.com/subscribe
You are currently unable to print this content. Please contact info@centralbanking.com to find out more.
You are currently unable to copy this content. Please contact info@centralbanking.com to find out more.
Copyright Infopro Digital Limited. All rights reserved.
As outlined in our terms and conditions, https://www.infopro-digital.com/terms-and-conditions/subscriptions/ (point 2.4), printing is limited to a single copy.
If you would like to purchase additional rights please email info@centralbanking.com
Copyright Infopro Digital Limited. All rights reserved.
You may share this content using our article tools. As outlined in our terms and conditions, https://www.infopro-digital.com/terms-and-conditions/subscriptions/ (clause 2.4), an Authorised User may only make one copy of the materials for their own personal use. You must also comply with the restrictions in clause 2.5.
If you would like to purchase additional rights please email info@centralbanking.com