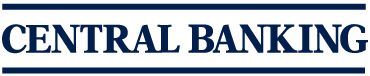
This article was paid for by a contributing third party.
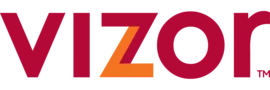
Suptech focus – AI to the rescue?

With the increased use of artificial intelligence in financial services, Vizor Software explains why it is crucial that regulators increase their knowledge of the potential risks while simultaneously raising internal AI skill levels and usage.
The interest in artificial intelligence (AI) has ballooned in recent years, with an unprecedented level of merger and acquisition activity from large tech firms in AI start-ups. Within the financial services industry, AI technology is becoming embedded in many consumer interactions and core business processes. You may have already experienced machine learning (ML) first hand when searching online for new financial products, with ‘robo-advice’ and customer recommendations suggesting what might suit you. Natural language processing (NLP) is commonly used by chatbots, in anti-money laundering, countering the financing of terrorism and fraud detection, and neural networks support many algorithmic trades. The use of AI is increasing and is here to stay.
AI is seen by many established financial institutions as an opportunity to reduce back-office costs and a necessity to stay competitive. With the word ‘tech’ appended to so many areas of the financial services industry to create new market categories for investors – such as regtech, fintech, insuretech and suptech – start-ups and technology giants see AI as a huge enabler for growth and disruption. As Ashish Gupta, chief technology officer (CTO) of Indian unicorn PolicyBazaar, says: “Our mandate is to grow 100% year-on-year for the next two to three years. But how do you do this by increasing manpower 30–40%? That’s where automation, intelligence and analytics helps.”
So what does AI mean for regulators? First, there is an expectation that regulators are aware of and are providing industry guidance on what ‘good’ looks like in the responsible use of AI. Second, regulators who are consuming increasing amounts of big data are starting to use AI to gain new insights and inform policy decisions. A 2017 report from the Bank for International Settlements indicated the number of central banks that incorporate big data analytics into policy-making and supervisory processes doubled, from 30% to 60%, in just two years.
For many regulators, a key challenge in assessing new AI risks and making best use of AI internally will be the significant upskilling of its staff. “With the rate of technology adoption snowballing, regulators have to rethink their approach and take time to build the resources and skills needed,” says Joanne Horgan, chief innovation officer at Vizor Software.
Leading regulators are already acting on both fronts. The Bank of England and the UK Financial Conduct Authority surveyed the industry in March 2019 to understand how and where AI and ML are being used, and early results indicate 80% of survey respondents are using ML.
In 2018, the Monetary Authority of Singapore released guidance about the responsible use of AI and moved their chief data officer, David Hardoon, to a new AI special adviser role focused on helping to develop the AI strategy for Singapore’s financial sector.
While many regulators are embracing AI, they are also acknowledging the risks. The Australian Prudential Regulation Authority’s Geoff Summerhayes says, aside from “opportunities that AI and ML present for fine-tuning and innovation in risk assessment, underwriting, loss prevention and customer engagement… algorithm use also brings risks that are not yet fully understood by [the] industry or regulators”.
Ryan Flood, CTO at Vizor Software, explains, up to now, “most regulators have focused on applying ML retrospectively to gain insights. Few have looked at embedding ML into front-line supervision, and we believe there is huge opportunity to supplement rules-based data checks with ML algorithms to highlight data anomalies and risks at the point of data collection.” While the existing method of predefining rules to increase data quality and perform plausibility checks undoubtedly increases the quality of data coming into the regulator, the increased appetite for granular and big data combined with the pace of regulatory change means a new approach is needed.
Step one to increasing the adoption of AI within the regulator and helping front-line supervisors become comfortable talking to their firms about AI is to increase staff engagement, Vizor’s Horgan says. “Embedding ML into the day-to-day job of supervisors and policy-makers, educating staff and demystifying it, is key.”
Big data is the bedrock of many predictive analytics implementations of ML where historical data is analysed to provide insight into future performance. It provides the volume, variety and velocity of data needed to identify patterns or find anomalies. Another important ‘V’ is the veracity or quality of the dataset being used to train the algorithms. If poor-quality or incomplete data is the starting point for your ML project, you need to first focus on improving the quality of that data.
An example showcasing the risks of ML is the story of Amazon.6 To improve efficiency of their HR job applicant screening process they had an algorithm rank the top 5 CVs. However, the training data used was a 10-year historical set of successful applicants (in the mostly male-dominated IT industry). The algorithm filtered out CVs with the word ‘women’, leading to unintended bias.
Are we suggesting regulators should clamp down on AI/ML because of its risks, or rush towards a future of automated regulation? Well, neither. With AI well on its way to ‘crossing the chasm’ from the innovators and early adopters into mainstream, we believe the risks of ML need to be understood as there is potential danger in relying solely on predictors of past failures. Equally, regulators should recognise ML to augment supervisory processes, not be a replacement for them.
Our advice, as Nike says, is “Just do it” – start small but with the big picture in mind and move fast without leaving people behind. Articulate a business problem where you think AI/ML can help, and be clear about the measurable outcome you hope to achieve, not just the output of having completed a proof of concept. Decide whether you want to start with building internal data science skills, gaining real insights to inform policy decisions or increasing the quality, speed and efficiency of supervisory processes.
Read the full Central Banking FinTech & RegTech Global Awards 2019 Winners In Focus report
Sponsored content
Copyright Infopro Digital Limited. All rights reserved.
As outlined in our terms and conditions, https://www.infopro-digital.com/terms-and-conditions/subscriptions/ (point 2.4), printing is limited to a single copy.
If you would like to purchase additional rights please email info@centralbanking.com
Copyright Infopro Digital Limited. All rights reserved.
You may share this content using our article tools. As outlined in our terms and conditions, https://www.infopro-digital.com/terms-and-conditions/subscriptions/ (clause 2.4), an Authorised User may only make one copy of the materials for their own personal use. You must also comply with the restrictions in clause 2.5.
If you would like to purchase additional rights please email info@centralbanking.com