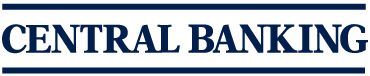
This article was paid for by a contributing third party.

Putting big data into action
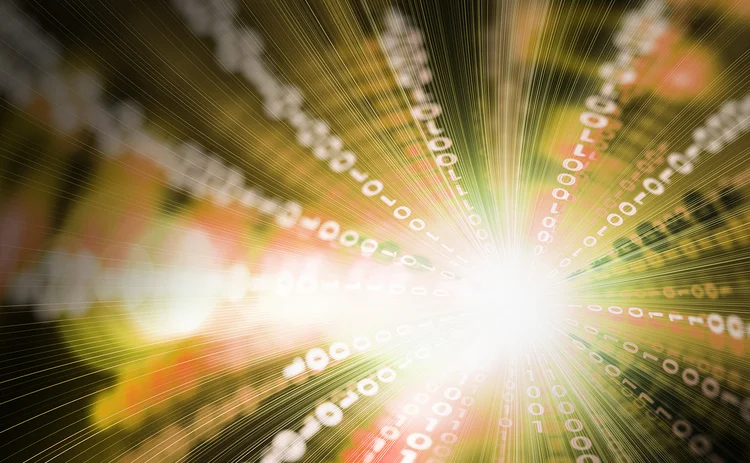
Big data is changing the way central banks think about the economy and oversee the financial system. In a forum sponsored by BearingPoint, Central Banking convened a panel of experts to discuss the innovative ways central banks are making use of big data.
The panel
- David Bholat, Senior Manager, Advanced Analytics, Bank of England
- Jyry Hokkanen, Head of Statistics, Sveriges Riksbank
- Moderator: Daniel Hinge, Report Editor, Central Banking
Supervisors and economists are increasingly being required to deal with large volumes of data – often unstructured and high frequency – day to day. Big data is therefore becoming an unavoidable fact of central bankers’ work as it matures as a field, and it is changing the way they think about the economy and oversee the financial system.
Our panel discusses how central banks can operationalise big data for purposes such as satisfying technological needs, resourcing, and bringing about organisational change. Ideas are raised on how central banks and other supervisors can maximise the potential of data while overcoming hurdles to its collection and deployment, as well as offering valuable insights for those working with data and who want to unlock the potential of this fast-growing field. The panel also explores examples of the innovative approaches central banks are taking to big data, and advising on establishing or building up a big data division within a central bank.
Central Banking: It means different things to different people, but how do you define big data?

David Bholat, Bank of England (BoE): The conventional definition is always around the ‘three Vs’ – big volume, fast velocity and a variety of data sources. In practical terms, it means you have very large, naturally numerical datasets of structured data – large enough that they don’t fit within an Excel spreadsheet – so you have to use a database or have some sort of underlying solution for that massive amount of granular numerical data. The other aspect is thinking about data that needs to be transformed in some way. For example, when text is your data there is a need to turn qualitative information into quantitative figures.
Jyry Hokkanen, Sveriges Riksbank: People look at big in very different ways. I think of it as structured and unstructured data, while most associate it with unstructured data – text, data from the internet, and so on. Many central banks also see structured data coming in large quantities. We are moving into more and more granular data, so these are large datasets that are reported with a high frequency to the central bank, and they are now analysed in different ways than before.
Central Banking: How is big data changing? Are we seeing more widespread adoption in central banks and the private sector?
David Bholat: Yes, unequivocally among both central banks and the broader financial sector. The reason is that you have both supply and demand factors at work. On the supply side there is an accumulation of data – from everyday uses and appliances such as mobile phones or Google searches – which is constantly being created, and therefore the opportunity exists to mine it in some way. Plus, you have the development of very cheap tools to store and analyse that data, and the development of cloud computing, which means organisations can have a lot more data storage capacity. On the other end of the data analytical spectrum are open-source tools like Python and R that come with ready-made machine-learning packages. Again, they’re free, so there’s a huge value proposition there.
On the demand side, both central banks and financial firms see the need to drive operational efficiency, particularly in the private sector among financial firms – to the extent that we are now in a low-interest environment and you can’t drive top-line growth. Margin can therefore only be maintained by cutting operational expenses.
Jyry Hokkanen: I completely agree – there’s so much data on the internet from economic agent activities that can be collected and stored cheaply. The question is: how is this interesting for central banks? It takes a lot of effort to analyse this data because it is so unstructured. We see a lot of interesting research on the unstructured side of big data, but it’s going to be difficult for central banks to use it in a meaningful way. Structured big data is more for central banks to analyse financial institutions and markets, and hopefully monetary policy as well to aid macroeconomic analysis.
Central Banking: How is Sveriges Riksbank making use of big data?
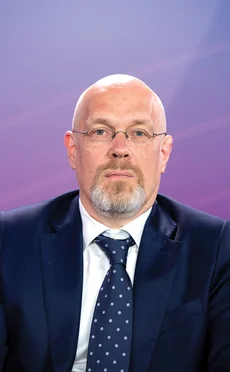
Jyry Hokkanen: Sveriges Riksbank has some projects based on individuals doing interesting research. Like many others, we have been scraping data from the web on prices, which has been quite promising. It’s very cheap and efficient, and has fed into our monetary policy process. Other research is on text mining, but it is tricky to show the stability of indicators of sentiment analysis. We are also looking at central banks’ speeches, of which the Bank for International Settlements has a database that can be analysed to determine whether central bank communication has any effect on financial marketplaces.
Central Banking: It is interesting that it is easier to extract meaning from a speech or an article than, for example, a
dialogue between two people.
Jyry Hokkanen: Exactly. We meet companies, survey them by interviewing and asking questions, but we also have a dialogue with them. After each dialogue, we used to analyse a transcript of the discussion, but that was really difficult because dialogue isn’t necessarily structured to contain a clear message, as an article or speech does, where you really emphasise the point. Dialogue touches on many different things that are still very difficult for a machine to analyse. That is also a problem with analysing Twitter, which we haven’t done but I understand others have.
David Bholat: The BoE has previously analysed Twitter, yes. In a previous Central Banking forum I mentioned a project undertaken ahead of the Scottish independence referendum vote, where we tried to determine whether social media could provide any sort of leading indicator of any potential retail runs on Scottish banks. One of the key words searched for in those tweets was ‘RBS’, but RBS can also be a abbreviation for ‘running backs’. If you are an American football fan, on Sundays a lot of people will be tweeting along the lines of “the running back gained a certain amount of yards” and there will be a sudden massive spike with RBS. So it is very noisy.
Central Banking: What new projects are the BoE currently working on?
David Bholat: Your typology of thinking about this as structured and unstructured data also very nicely corresponds to the comparison I was drawing between text as data and data that is naturally numerical. To provide some examples: first, on text as data, we have a really interesting project where the researcher in my team has taken data from millions of job vacancies on one of the leading employment websites in the UK. The reason for looking at this information is that the UK Office for National Statistics – as with many other national statistics agencies – generates great statistics on the number of people in employment, in what sectors they are employed, what industries, and so forth. But where we have less insight is on the supply of jobs being offered. Looking at job vacancy data can actually provide a sense of how the productive structure of the economy is changing by the types of jobs being advertised.
The researcher generated a very granular view of the labour market from the bottom up using a latent Dirichlet allocation topic model – an unsupervised machine-learning clustering approach that allows the user to create job classification schemes as they emerge from data rather than, as is traditional, imposing a top-down classification. That can be important as, if the nature of work and the labour market is changing, you don’t necessarily want to rely on a pre-existing industrial classification scheme to tell you what jobs people are in, but rather to see how new jobs are emerging.
For the analysis of very large, granular, naturally numerical datasets, in the UK we have a dataset called product sales data, which contains a record of every single regulated mortgage originated in the UK since 2005 at loan level. This includes information at origination, but also subsequent performance data. The analysts looked at this data to identify the stock of currently outstanding mortgages that are high risk. By looking at that data we could see that, in contradistinction to surveys suggesting those high-risk mortgages were rapidly falling off, it looked more like things were flatlining. That is the difference between taking a look at the data in full versus just survey results.
Central Banking: The Prudential Regulation Authority (PRA) is also using big data. Is that in textual analysis?
David Bholat: Indeed. Even in insurance, where we had the Solvency II regulation, which means insurers are having to report very granular data on an asset-by-asset line item basis. We’re also looking internally at how we can use text-mining approaches to better understand the complexity of regulation. We have an interesting project where we’re looking at the PRA rulebook to try to understand how complex it is, where complexity is a function of structural complexity, how long it is, how interconnected it is in terms of citational links and thinking of web pages as nodes in a network, as well as linguistic complexity – how readable our regulations are in terms of standard readability metrics.
Jyry Hokkanen: On mortgage rates, in Europe we’re going to have AnaCredit – which is mandatory for the eurozone and voluntary for the other European Union member states – which Sweden is going to join. Then we are going to have loan-by-loan data with a lot of information on each loan. The same kind of analysis as previously discussed will be undertaken by many European central banks.
David Bholat: That is an important point; all of these advanced analytic techniques really presuppose you have the data. You need to sort out the meat and potatoes first, and then move on to dessert.
Central Banking: Is that data a challenge? Do you spend a lot of time cleaning, organising and preparing data?
David Bholat: Absolutely. I would say probably 50% of a data scientist’s job is trying to clean up the datasets, whether naturally numerical or text‑based. Obviously if it’s text there’s a whole process by which you need to convert those words into numbers prior to doing the analysis, but even with regulatory return data you have to perform validity checks and possibly infer missing values, and often the population of firms reporting changes over time.
Central Banking: Is it difficult to automate much of that work – does it require a lot of hard work by data scientists?
David Bholat: It does, but I think that’s where you see investment, certainly in industry and among central banks is how we can automate what are traditionally routine or very manual processes in the data pipeline – which is where a lot of energy is focused at present.
Jyry Hokkanen: We’re going to employ our first data scientist this summer, so we have been thinking about what a data scientist should do. The first thing is to handle and clean up the data, and we will primarily be working with structured loan-by-loan, security-by-security data.
Central Banking: The BoE was certainly quick off the mark in establishing its Advanced Analytics Division. Has your experience been one of support from senior managers?
David Bholat: For large transformation projects, you need executive buy-in. We certainly had that four years ago when Advanced Analytics was set up. The encouraging sign has been that, aside from having buy‑in from the top, we’ve also got a wider data ecosystem emerging. Advanced Analytics is only one part of that ecosystem within the bank – we also have a chief data officer (CDO) division, and their responsibility is to create the infrastructure, which I earlier called the meat and potatoes, that can serve everybody within the organisation.
There are also lots of people using machine-learning techniques for different purposes. Over time it won’t be about having a particular executive champion or area leading on data science or big data, but rather it will become ‘business as usual’ – these will just be tools and techniques that are part of the wider infrastructure that central banks use as a matter of course.
Central Banking: Is the first catalyst to showing that you’re interested in building the data infrastructure appointing a CDO or similar executive-level position to send a signal?
David Bholat: That’s part of it, but you really need a champion among your governors. The governor has to buy in to it, you need buy-in from the very top.
Jyry Hokkanen: You also need outside pressure and role models – other central banks that you look up to, such as the BoE or the US Federal Reserve.
Central Banking: Is it a challenge to hire data scientists, especially ones with economics backgrounds?
David Bholat: The BoE has managed this, but we haven’t just hired economists who have completed data science courses. The majority of people who work within the division aren’t economists – they’re physicists, mathematicians, computer scientists, and there’s even an experimental psychologist and a linguist. Multidisciplinary teams have been shown to approach problems differently and bring different perspectives, thereby problem-solving in a more creative way than with a homogenous team. But it does mean that that our area has a relative lack of central banking experience and economics knowledge. We’ve remedied that by partnering very closely with business areas.
Central Banking: A Central Banking survey in 2017 found that 68% of central banks were working on new big data projects. Of these, 36% viewed big data as a core input policy – do you find this surprising?
David Bholat: I don’t know if I am particularly surprised. It’s an emerging field, and five years ago big data was still a topic not really being addressed by the central banking community. Now it very much is.
Central Banking: Post-financial crisis, the quantities of banking data, banking regularity data, derivatives data, and so on, almost forced central banks to handle big data by default. What are the drawbacks to big data? Could this replace other forms of analysis?
David Bholat: Definitely not, and my advice to any central banking colleagues would be to avoid novelty for the sake of it. You need to have a good business case for big data or you won’t gain any traction. You need to be addressing a policy-relevant issue for which a big data source might exist that can be exploited.
Jyry Hokkanen: You should be curious, look into new techniques, what others are doing and why they are doing it. If you already have the data in-house, then you should try to think about using this information optimally – does it correlate with something else you are interested in, and how can you exploit that?
Central Banking: What are the main checkpoints that the BoE goes through before implementing big data projects?
David Bholat: It varies from project to project. In terms of our process for setting up an area such as Advanced Analytics, we didn’t go straight away to a productionised solution – we began with pilots. We started out with a small vision team hired specifically to think about what the data strategy should be for the organisation post-financial crisis, and then reflected on what was occurring in the wider economy. We concluded that it would be best to move towards using big data and collecting data at a more granular level.
Central Banking: Something that came from the survey was that central banks tend to build their data platforms in-house. Is this the case at the BoE?
David Bholat: The model we’ve evolved is a mixture of buy and build, so some of the things we’ve done have been built in-house, but a lot of it – particularly hardware solutions – has been purchased externally.
Central Banking: Do you use cloud services?
David Bholat: Not yet, but we’re about to – we’re in the process of undertaking a project on the cloud. It’s coming, but it’s still an area that we haven’t settled on in terms of whether it will be a productionised solution or not, or whether it will just be contained to a particular pilot.
Jyry Hokkanen: Most central banks are reluctant to use cloud services for security reasons, so that will be a big obstacle, but if it can be overcome then suddenly everybody who knows these things will say ‘this is cheap and efficient’.
Central Banking: What are the key big data research questions – what needs to be answered and what would you like answered in the next few years?
Jyry Hokkanen: I like text-mining techniques. I’d like that to become stable, because there’s huge potential in it. We do communicate and central banks produce text, but we also receive a lot of text, and you can monitor society and the economy via tweets, speeches, articles and company statements.
David Bholat: BoE recently completed a project where we text-mined the letters our supervisors write to the firms they regulate. We then used a machine-learning algorithm called a random forest model to see whether we systematically write to firms differently depending on whether they are large or small and, importantly, whether we’re writing to firms – and therefore supervising them – differently. Voice-to-text, then text-to-data technologies could also be massively labour-saving for bank supervisors. Our supervisors spend a lot of time speaking to firms and taking notes. If you deployed voice-to-text technology and had verbatim transcripts that were automatically logged as text, you could do quantitative analysis.
Watch the full proceedings of the Central Banking BearingPoint forum, Putting big data into action.
Sponsored content
Copyright Infopro Digital Limited. All rights reserved.
As outlined in our terms and conditions, https://www.infopro-digital.com/terms-and-conditions/subscriptions/ (point 2.4), printing is limited to a single copy.
If you would like to purchase additional rights please email info@centralbanking.com
Copyright Infopro Digital Limited. All rights reserved.
You may share this content using our article tools. As outlined in our terms and conditions, https://www.infopro-digital.com/terms-and-conditions/subscriptions/ (clause 2.4), an Authorised User may only make one copy of the materials for their own personal use. You must also comply with the restrictions in clause 2.5.
If you would like to purchase additional rights please email info@centralbanking.com