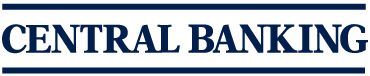
This article was paid for by a contributing third party.

Insights from network analytics in suptech
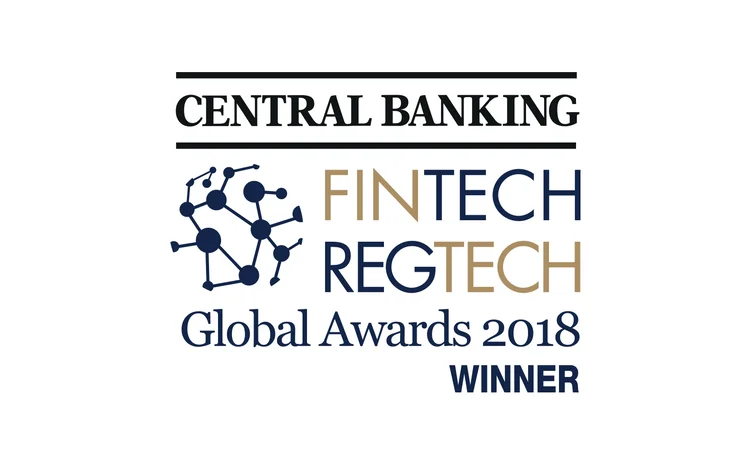
With regulators leveraging technological innovations to move towards informed, data‑driven decision‑making and automation, supervisory technology is attracting enhanced interest. Kimmo Soramäki and Phillip Straley examine how regulators are gaining insight by examining interconnectedness in datasets, and how this can reduce risk in the financial system.
With the emergence of supervisory technology – often referred to as ‘SupTech’ – financial authorities are investigating new approaches to big data analytics. This article explores real‑world examples of insights regulators are gaining by examining interconnectedness in datasets, and how this can have a practical impact on reducing risk in the financial system. Financial Network Analytics (FNA) believes adoption of network analytics is at an inflection point. The supervisory marketplace increasingly realises that previously commonplace approaches to understanding financial institutions and market behaviour, while still valuable, are no longer sufficient.
What is driving suptech?
The SupTech domain is receiving increased attention from central banks and other supervisors globally for a number of reasons. One driver is that financial authorities are seeking to leverage the same technological innovations as commercial institutions, including data analytics, artificial intelligence and machine learning. In some markets, central banks have explicit market development objectives, and so need to push innovation. However, even where this is not the case, financial authorities understand the need to co-operate with the commercial market for efficiency – for instance, through more effective data collection and sharing mechanisms. But the most important reason we see SupTech gaining attention is simple: in an increasingly interconnected global financial marketplace, there is a broader and faster propagation of risk, requiring supervisors to have the tools to keep pace. Financial authorities want to move towards more informed, data‑driven decision‑making, and automating their own manual processes.1
Supervisory focus on analytics
In our experience of working with financial authorities, it is now common to find a centralised hub for analytics and related data management. Historically, pockets of analytics capability sat in myriad parts of a financial authority – in research and statistics, monetary policy, market supervision, financial crime units and macro surveillance – and such groups will continue to maintain their individual analytics competencies. However, as financial authorities look to keep pace and do more with limited resources, shared‑service analytics functions are a priority. Analytics tools are also undergoing rationalisation and enhancement.
The importance of network analytics and simulation
A key lesson from the 2007–08 global financial crisis was that, despite the deployment by supervisors and financial institutions of advanced analytics models – statistical and stress-based, among others – they did not provide an understanding of how risk might propagate across the complex global financial system, in terms of liquidity impacts, counterparty relationships and other market connections. Network analytics and related visualisation methods now offer financial authorities – and some market participants – increased understanding of the degree and meaning of these interconnections.
Application of network analytics and simulation in SupTech
Senior executives at global central banks and regulators in FNA workshops often list more than a dozen areas to which network analytics can be applied within their organisations. These are in areas as diverse as banking supervision, financial market infrastructure (FMI) design, oversight and operations, systemic risk surveillance, and financial crime surveillance. FNA undertakes extensive research and offers a number of solutions that address these areas for regulatory and supervisory clients.
Banking supervision
Individual regulators’ internal structures for financial institution supervision differ, but they usually combine supervision by type of institution – for example, domestic and international banks, systemically important banks and FMIs – with supervision by type of activity or risk – for example, credit risk, traded markets and financial crime compliance. Regulators adopt network approaches across all of these areas. Factors driving adoption of network analytics include the following:
- The need to integrate multiple data sources to understand – and visualise –participants’ interrelationships.
- The need to utilise granular data – for instance, individual transactions in payments systems – for non‑traditional uses from a supervision perspective.
- The need to look at multiple factors in understanding interconnectedness among market institutions.
- The need to model and stress‑test risk propagation across these networks.

FMI design, oversight and operation
FNA clients are involved in efforts to upgrade existing payment systems worldwide, including the multiyear modernisation project by Payments Canada, the Bank of England’s endeavours to modernise its Clearing House Automated Payment System, and the many initiatives providing new schemes for international payment settlement currently carried out by banks’ correspondent networks. These efforts are largely driven by the need to save liquidity in a post‑quantitative-easing era and meet customers’ increasing demands for faster payments.
Simulations have been used for the past 20 years to better understand the complex dynamics taking place in FMIs since the launch of the Bank of Finland Payment Systems Simulator in 1998. Simulations can provide a laboratory setting in which probable effects of different system designs and assumptions on payment flows or available liquidity can be analysed. Importantly, simulation models can be built to closely replicate the actual operating environment, or test and observe scenarios not normally found in historical operating environments. Simulations can also be carried out to study different crisis scenarios and how to best prepare for and mitigate them.
Finally, the Principles for Financial Market Infrastructures stipulate that systemically important FMIs must be able to complete settlement in the case of the failure of the largest – and, for the most important ones, the two largest – members. A natural way to demonstrate this is via simulations, where the liquidity displacement and settlement performance is tested by an artificial failure of such members. Simulations also help design remediation strategies and prepare for the impacts of a failure to avoid vicious cycles where the initial failure causes a cascade of new issues.
FNA research on central counterparty interconnectedness
In the wake of the 2007–08 financial crisis, global regulators moved to shift a growing portion of over-the-counter derivatives transactions onto central counterparty (CCP) clearing houses. This reduced bilateral counterparty risk but also substantially concentrated risk, making improved risk management imperative for CCPs. It has also increased the necessity for CCPs’ regulators to better and more fully understand the risks run by these CCPs.
FNA has worked with global CCPs, including one of the largest global derivatives exchanges, to apply network analytics to model relationships between their members and understand risk concentrations. This work has also included modelling the potential impacts of the failure of one or more significant settlement members to help design risk mitigation mechanisms for the CCP and its members.

Beyond this, FNA has recently undertaken fundamental analysis of interconnectedness across a number of the world’s major CCPs.2 Figure 1 illustrates, very broadly, the extreme interconnectivity through which shocks can propagate globally.
Thanks to internationally agreed-upon risk management standards and regular stress testing, the insolvency of a CCP is generally considered unlikely. A more likely scenario is the default of one of the clearing members of a CCP, with potential knock‑on effects within and across CCPs. Furthermore, although CCPs have strong risk controls in place to prevent insolvency, the liquidity and operational risks remain. In the event of an operational disruption or liquidity shortage within a CCP, its members would be affected, potentially impacting other CCPs that its members are associated with, and then impacting their members. Given this, contagion in the network as defined by CCPs and clearing members could potentially affect a large portion of the financial system, even in the absence of a CCP’s insolvency.
Anti‑money laundering and anomaly detection
With aggregate misconduct‑related fines of more than $350 billion since the financial crisis – a large portion of which is due to financial crime compliance lapses – addressing this area is important for financial institutions and supervisors. Furthermore, current methods for identifying money laundering are inadequate and costly in terms of the large amount of manual labour required, and continue to bloat financial institution – and regulatory – financial crime teams.
The challenge in anti-money laundering detection and investigation often involves mapping networks combining several data sources and identifying suspicious patterns – many of which can be automatically detected with network and graph algorithms. In many cases these networks are created manually, and large efficiency gains can be obtained by automating these processes. Once suspicious patterns are detected, often a manual investigation process begins. Insightful visual graph dashboards can then assist in investigation and prioritising cases more likely to prove problematic. Visual network dashboards allow case officers to overlay several relevant datasets to filter out noise – such as irrelevant clusters or low-risk accounts – and to recognise money laundering faster and with less effort.
About the authors
Kimmo Soramäki is the founder and chief executive of Financial Network Analytics (FNA), and Phillip Straley is FNA’s president. FNA is a deep technology company based in London and the first company worldwide dedicated to applying network theory in finance. FNA is trusted by many leading central banks, regulators and financial market infrastructure providers for analytics, artificial intelligence and machine-learning needs.
Notes
1. Official sector overviews have recently been published on the SupTech market, including Innovative technology in financial supervision (SupTech) – the experience of early users by the Bank for International Settlements (BIS), and From spreadsheets to SupTech: Technology solutions for market conduct supervision by the World Bank.
2. A BIS study using anonymised data was also published in July 2017 on this topic, entitled Analysis of central clearing interdependencies.
Read the full Central Banking FinTech RegTech Global Awards 2018 Winners In Focus report
Sponsored content
Copyright Infopro Digital Limited. All rights reserved.
As outlined in our terms and conditions, https://www.infopro-digital.com/terms-and-conditions/subscriptions/ (point 2.4), printing is limited to a single copy.
If you would like to purchase additional rights please email info@centralbanking.com
Copyright Infopro Digital Limited. All rights reserved.
You may share this content using our article tools. As outlined in our terms and conditions, https://www.infopro-digital.com/terms-and-conditions/subscriptions/ (clause 2.4), an Authorised User may only make one copy of the materials for their own personal use. You must also comply with the restrictions in clause 2.5.
If you would like to purchase additional rights please email info@centralbanking.com